ISIT 2018 Tutorial on Submodularity
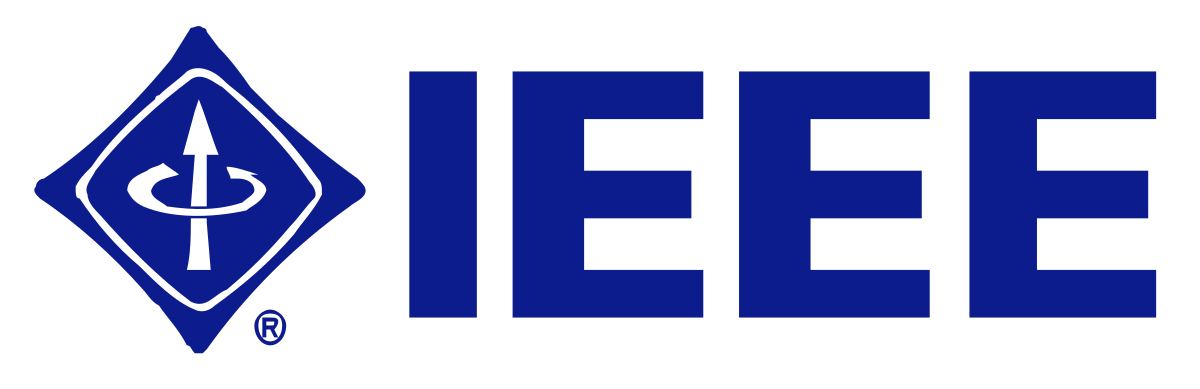
ISIT 2018 Tutorial on Submodularity in Information and Data Science.
Submodularity is a structural property over functions that has received significant attention in the mathematics community, owing to their natural and wide ranging applicability. In particular, numerous challenging problems in information theory, machine learning, and artificial intelligence rely on optimization techniques for which submodularity is key to solving them efficiently.We will start by defining submodularity and polymatroidality — we will survey a surprisingly diverse set of functions that are submodular and operations that preserve submodularity. Next we look at submodular functions that have been classically used as information measures and their novel applications in ML and AI including active/semi-supervised learning, structured sparsity, data summarization, and combinatorial independence and generalized entropy. Subsequently, we’ll define the submodular polytope, and its relationship to the various greedy algorithms and its exact and efficient solution to certain linear programs. We will see how submodularity shares certain properties with convexity (efficient minimization, discrete separation, subdifferentials, lattices and sub-lattices, and the convexity of the Lovasz extension), concavity (via its definition, submodularity via concave functions, superdifferentials), and neither (simultaneous suband super-differentials, efficient approximate maximization). Finally, we will discuss some extensions of submodularity that have proven useful in recent years.