Online Learning
In many practical applications, the environment is so complex that it may be infeasible to lay out a precise model and use the classical mathematical optimization methods. It is then necessary, and very often beneficial, to consider a robust approach, by considering optimization as a process that learns from experience as more aspects of the problem are being observed. This view of optimization as a process has become prominent in various fields and led to many successes in modeling and systems. One important research direction of our group is to study online learning in various settings, such as convex and submodular utility functions, and subject to full or partial information.
-
ICML 2023 Approximate Thompson Sampling with Logarithmic Batches: Bandits and Reinforcement Learning Amin Karbasi, Nikki Lijing Kuang, Yian Ma, Siddharth Mitra -
ICML 2023 Statistical Indistinguishability of Learning Algorithms Alkis Kalavasis, Amin Karbasi, Shay Moran, Grigoris Velegkas -
NeurIPS 2023 Replicability in Reinforcement Learning Amin Karbasi, Grigoris Velegkas, Lin F. Yang, Felix Zhou -
NeurIPS 2022 Black-Box Generalization Konstantinos E. Nikolakakis, Farzin Haddadpour, Dionysios S. Kalogerias, Amin Karbasi -
NeurIPS 2022 Fast Neural Kernel Embeddings for General Activations Insu Han, Amir Zandieh, Jaehoon Lee, Roman Novak, Lechao Xiao, Amin Karbasi -
NeurIPS 2022 Multiclass Learnability Beyond the PAC Framework: Universal Rates and Partial Concept Classes Alkis Kalavasis, Grigoris Velegkas, Amin Karbasi -
NeurIPS 2022 On Optimal Learning Under Targeted Data Poisoning | Oral Presentation Idan Mehalel, Steve Hanneke, Shay Moran, Mohammad Mahmoody, Amin Karbasi -
NeurIPS 2022 The Best of Both Worlds: Reinforcement Learning with Logarithmic Regret and Policy Switches Grigoris Velegkas, Zhuoran Yang, Amin Karbasi -
NeurIPS 2022 Universal Rates for Interactive Learning | Oral Presentation Steve Hanneke, Amin Karbasi, Shay Moran, Grigoris Velegkas -
NeurIPS 2020 Minimax Regret of Switching-Constrained Online Convex Optimization: No Phase Transition Lin Chen, Qian Yu, Hannah Lawrence, Amin Karbasi -
NeurIPS 2020 Online MAP Inference of Determinantal Point Processes Aditya Bhaskara, Amin Karbasi, Silvio Lattanzi, Morteza Zadimoghaddam -
PhD Thesis 2020 Online Optimization: Convex and Submodular Functions Lin Chen and Amin Karbasi -
AISTATS 2019 Projection-Free Bandit Convex Optimization Lin Chen, Mingrui Zhang, Amin Karbas -
arXiv 2019 Batched Multi-Armed Bandits with Optimal Regret Hossein Esfandiari, Amin Karbasi, Abbas Mehrabian, Vahab S. Mirrokni -
arXiv 2019 Minimax Regret of Switching-Constrained Online Convex Optimization: No Phase Transition Lin Chen, Qian Yu, Hannah Lawrence, Amin Karbasi -
NeurIPS 2019 Online Continuous Submodular Maximization: From Full-Information to Bandit Feedback Mingrui Zhang, Lin Chen, Hamed Hassani, Amin Karbasi -
AISTATS 2018 Online Continuous Submodular Maximization | Oral Presentation Lin Chen, Hamed Hassani, Amin Karbasi -
ICML 2018 Projection-Free Online Optimization with Stochastic Gradient: From Convexity to Submodularity. Lin Chen, Christopher Harshaw, Hamed Hassani, Amin Karbasi -
AISTATS 2014 Near Optimal Bayesian Active Learning for Decision Making Shervin Javdani, Yuxin Chen, Amin Karbasi, Andreas Krause, Drew Bagnell, Siddhartha S. Srinivasa:
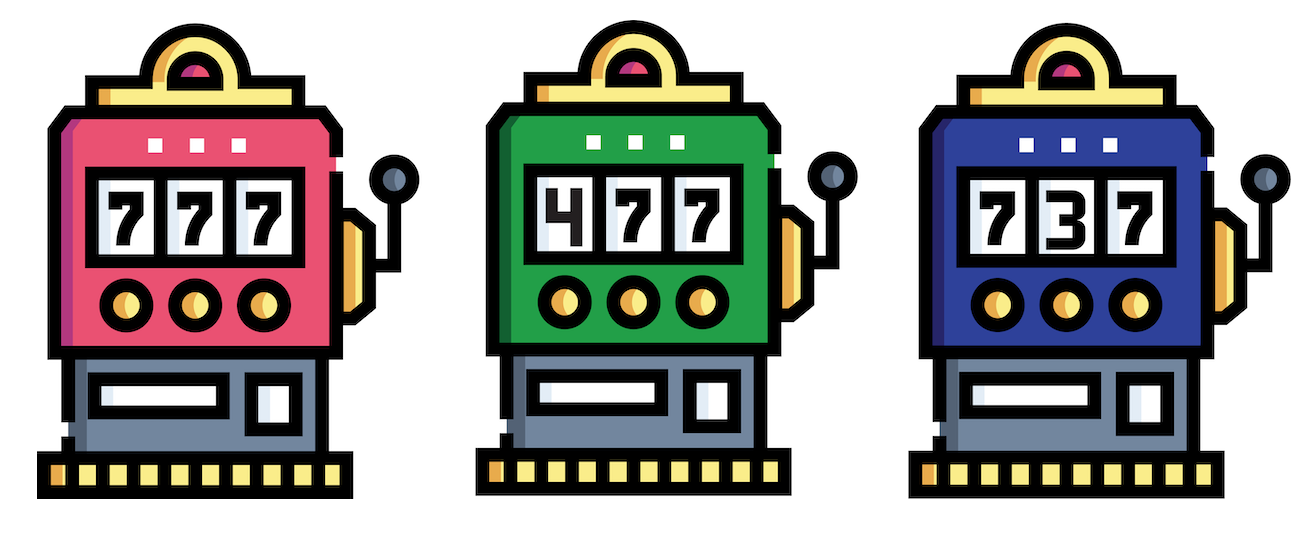