Trustworthy ML & Robust Statistics
As machine learning systems are pervasively deployed in many scientific fields with increasingly sensitive tasks, it has become paramount to develop algorithms that are robust against the numerous sources of uncertainty inherent in those applications including noise in the data, malicious exploitation of vulnerabilities, outliers, variability of the true objective, privacy, and fairness. While current research in machine learning has led to fundamental breakthroughs, there is still a large gap between the theory and the limitations of the existing algorithms used by practitioners in the real world. An important research direction for our group is to lift the current methods out of the sterile lab environment and scale them into the messy real world, by carefully reexamining their limitations, considering more realistic but less perfect conditions, and developing correspondingly robust algorithms.
-
AISTATS 2022 Federated Functional Gradient Boosting Zebang Shen, Hamed Hassani, Satyen Kale, Amin Karbasi -
ICLR 2022 Learning Distributionally Robust Models at Scale via Composite Optimization Farzin Haddadpour, Mohammad Mahdi Kamani, Mehrdad Mahdavi, and Amin Karbasi -
ICLR 2022 Scalable Sampling for Nonsymmetric Determinantal Point Processes | Spotlight Insu Han, Mike Gartrell, Jennifer Gillenwater, Elvis Dohmatob, Amin Karbasi -
AAAI 2021 Regret Bounds for Batched Bandits Hossein Esfandiari, Amin Karbasi, Abbas Mehrabian, Vahab Mirrokni -
AISTATS 2021 Meta Learning in the Continuous Time Limit Ruitu Xu, Lin Chen, Amin Karbasi -
arXiv 2021 Batched Neural Bandits Quanquan Gu, Amin Karbasi, Khashayar Khosravi, Vahab Mirrokni, Dongruo Zhou -
UAI 2021 Learning and Certification under Instance-targeted Poisoning Ji Gao, Amin Karbasi, Mohammad Mahmoody -
UAI 2021 The curious case of adversarially robust models: More data can help, double descend, or hurt generalization Yifei Min, Lin Chen, Amin Karbasi -
AISTATS 2020 Black Box Submodular Maximization: Discrete and Continuous Settings Lin Chen, Mingrui Zhang, Hamed Hassani, Amin Karbasi: -
AISTATS 2020 Quantized frank-wolfe: Faster optimization, lower communication, and projection free Mingrui Zhang, Lin Chen, Aryan Mokhtari, Hamed Hassani, Amin Karbasi -
arxiv 2020 Safe Learning under Uncertain Objectives and Constraints Mohammad Fereydounian, Zebang Shen, Aryan Mokhtari, Amin Karbasi, Hamed Hassani -
ICML 2020 More data can expand the generalization gap between adversarially robust and standard models Lin Chen, Yifei Min, Mingrui Zhang, Amin Karbasi -
AAAI 2019 Eliminating Latent Discrimination: Train Then Mask Soheil Ghili, Ehsan Kazemi, Amin Karbasi -
AISTATS 2018 Comparison Based Learning from Weak Oracles Ehsan Kazemi, Lin Chen, Sanjoy Dasgupta, Amin Karbasi -
ICML 2018 Scalable Deletion-Robust Submodular Maximization: Data Summarization with Privacy and Fairness Constraints. Ehsan Kazemi, Morteza Zadimoghaddam, Amin Karbasi -
ICML 2018 Weakly Submodular Maximization Beyond Cardinality Constraints: Does Randomization Help Greedy? Lin Chen, Moran Feldman, Amin Karbasi -
IEEE/ACM Transactions on Networking 2013 Robust Localization From Incomplete Local Information Amin Karbasi, Sewoong Oh -
IEEE Transactions on Information Theory 2012 Graph-Constrained Group Testing Mahdi Cheraghchi, Amin Karbasi, Soheil Mohajer, Venkatesh Saligrama -
ISIT 2010 Graph-Constrained Group Testing | Best Student Paper Award (runner up) Mahdi Cheraghchi, Amin Karbasi, Soheil Mohajer, Venkatesh Saligrama -
Alerton 2009 Compressed Sensing with Probabilistic Measurements: A Group Testing Solution Mahdi Cheraghchi, Ali Hormati, Amin Karbasi, Martin Vetterli: -
arXiv 2009 An Estimation Theoretic Approach for Sparsity Pattern Recovery in the Noisy Setting Ali Hormati, Amin Karbasi, Soheil Mohajer, Martin Vetterli -
ISIT 2009 Support recovery in compressed sensing: An estimation theoretic approach Amin Karbasi, Ali Hormati, Soheil Mohajer, Martin Vetterli:
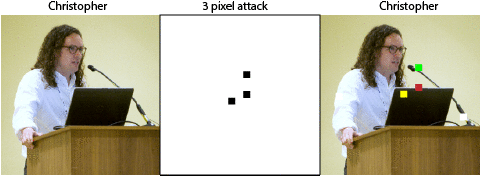