Non-convex Optimization
Until recently, convex programs were seen as the defining boundary for tractability in continuous optimization. However, many problems of interest arising from machine learning and statistical modeling, such as training deep neural networks and learning latent variable models, are glaringly non-convex. While efficient algorithms are known for a few instances of non-convex problems, it remains a central challenge to discover general conditions under which a non-convex problem admits an efficient solution.
-
ICML 2023 Accelerating Transformers via Kernel Density Estimation Amir Zandieh, Insu Han, Majid Daliri, Amin Karbasi -
NeurIPS 2023 Optimal Guarantees for Algorithmic Reproducibility and Gradient Complexity in Convex Optimization | Spotlight Presentation Liang Zhang, Junchi YANG, Amin Karbasi, Niao He -
NeurIPS 2023 Optimal Learners for Realizable Regression:PAC Learning and Online Learning | Oral Presentation Idan Attias, Steve Hanneke, Alkis Kalavasis, Amin Karbasi, Grigoris Velegkas -
NeurIPS 2023 Replicable Clustering Hossein Esfandiari, Amin Karbasi, Vahab Mirrokni, Grigoris Velegkas, Felix Zhou -
AISTATS 2020 Black Box Submodular Maximization: Discrete and Continuous Settings Lin Chen, Mingrui Zhang, Hamed Hassani, Amin Karbasi: -
AISTATS 2020 One Sample Stochastic Frank-Wolfe Mingrui Zhang, Zebang Shen, Aryan Mokhtari, Hamed Hassani, Amin Karbasi -
Journal of Machine Learning Research 2020 Stochastic Conditional Gradient Methods: From Convex Minimization to Submodular Maximization Aryan Mokhtari, Hamed Hassani, Amin Karbasi -
NeurIPS 2020 Continuous Submodular Maximization: Beyond DR-Submodularity Moran Feldman, Amin Karbasi -
PhD Thesis 2020 Online Optimization: Convex and Submodular Functions Lin Chen and Amin Karbasi -
SIAM Journal on Optimization 2020 Stochastic Conditional Gradient++ Hamed Hassani, Amin Karbasi, Aryan Mokhtari, Zebang Shen -
NeurIPS 2019 Stochastic Continuous Greedy ++: When Upper and Lower Bounds Match Amin Karbasi, Hamed Hassani, Aryan Mokhtari, Zebang Shen -
STOC 2019 Unconstrained submodular maximization with constant adaptive complexity Lin Chen, Moran Feldman, Amin Karbasi -
AISTATS 2018 Conditional Gradient Method for Stochastic Submodular Maximization: Closing the Gap Aryan Mokhtari, Hamed Hassani, Amin Karbasi -
AISTATS 2018 Submodularity on Hypergraphs: From Sets to Sequences Marko Mitrovic, Ehsan Kazemi, Morteza Zadimoghaddam, Amin Karbasi -
ICML 2018 Data Summarization at Scale: A Two-Stage Submodular Approach M . Mitrovic, E. Kazemi, M. Zadimoghaddam, A. Karbasi -
ICML 2018 Decentralized Submodular Maximization: Bridging Discrete and Continuous Settings. Aryan Mokhtari, Hamed Hassani, Amin Karbasi:
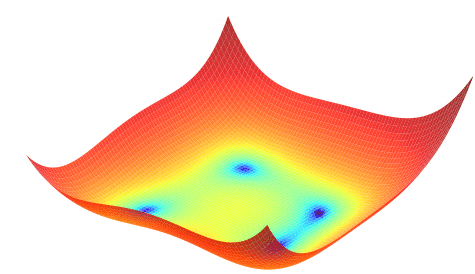